Expert Insight: Chris Gotwalt (JMP) on Text Explorer in JMP 13
JMP's Director of Statistical R&D discusses the new Text Explorer tool in JMP 13.
We recently joined forces with statistical software company JMP to co-host a special seminar titled Increasing the Value of your Experiments with Enhanced Analytics. Presented by JMP’s Director of Statistical Research and Development Chris Gotwalt, the event explored the power tools available to scientists, engineers and formulators for analysing Design of Experiments data sets, and highlighted how to maximise what you learn from experiments by using enhanced modelling.
Following the presentation, Chris took questions from the floor about wider statistical methodologies. With JMP 13 now released, it gave those attending the chance to quiz the software developer about what’s new in the latest version.
One topic which caused a lot of excitement was a discussion about text analysis and the arrival of a Text Explorer platform in JMP. Here we learn from Chris exactly what JMP users can expect and how best to use the software.
Chris Gotwalt on... the addition of text analysis to the new version of JMP:
There’s a lot of text data out there, but prior to the release of JMP 13 there wasn’t a seamless way to analyse text data in JMP. We’ve been seeing freeware tools pop up and people have been beginning to develop stand-alone JSL add-ins to get data into JMP, which then enables them to do text processing and analysis within JMP. After having watched this unfold over a couple of releases, we went ahead and added a native text analysis platform in version 13 to make it much easier to analyse that kind of data.
Chris Gotwalt on... Text Explorer being available in both JMP 13 and JMP Pro 13:
There are two aspects to text analytics. There’s the data munging phase where you’re converting the text data into what’s called the document term matrix, which is like a multivariate representation of the text. In order to do that, you need to be able to address misspellings, you have to apply stemming rules to make sure that different conjugations of the same word are treated as the same, you have to handle regular expressions in order to do more automated and sophisticated transformations of your data, and you must determine what phrases to add, identify ‘stop’ words… all this infrastructure around the basic processing of text data! That component is available in JMP 13.
Once you’ve got that set up, then there are a variety of analytic tools you can use to do clustering and dimension reduction – using an SVD approach to reduce the number of vectors that require attention and help build regression formulas. You have scoring, theming… all these kinds of analytical tools. We have developed text-specific algorithms to analyse text data using these kind of techniques. What happens when you do text analysis is that you often end up with loads of rows, because it’s easy to generate text. The conversion is usually: ‘take in the text data and turn it into a design matrix’ where you have indicators for whether a term or phrase was present in a particular document – but, you know, how many words are there in the English language? The potential number of single word columns in this matrix alone becomes very, very wide. We identified that processing that part of the data is a ‘big data’ problem, and so when my team and I were developing the algorithms for it we recommended that this be part of JMP Pro as it’s more in line with the Pro vision.
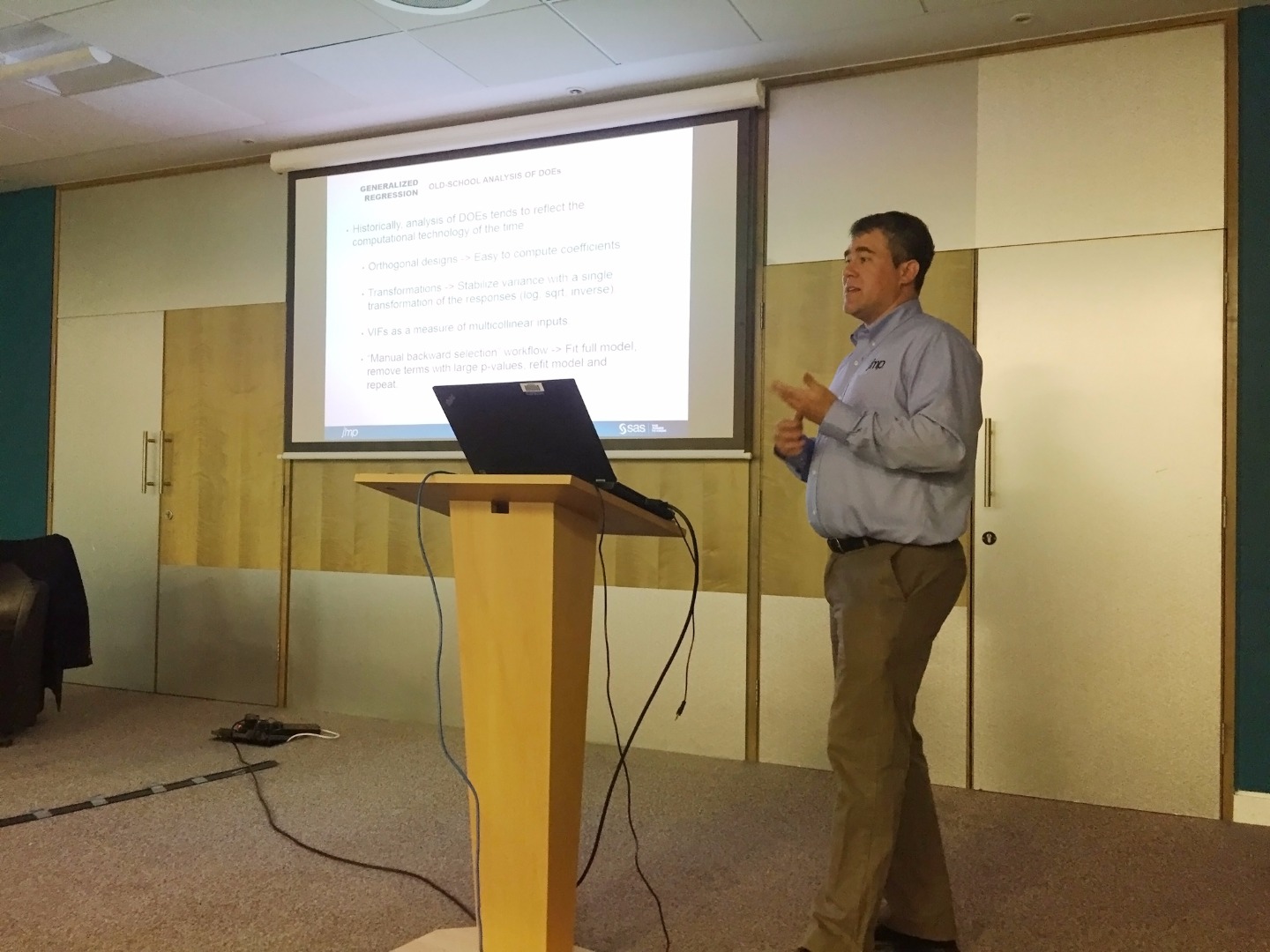
Chris Gotwalt on... how to do text analysis within JMP:
I have a text data set with a bunch of airplane crash reports from the United States over the course of two years. We have some information about the location, the damage that was done, the kind of aircraft that was involved in the accident and whether the accident was fatal or not. Also, the National Transport Safety Board (NTSB) requires that there is a write-up of what happened. We have narratives which describe what has taken place. So we can process them using the text platform pretty easily – in JMP 13 we have this new Text Explorer, which is at the top of the Analyse menu. We put it here because we believe there’s lots of text data out there and people are going to be quite interested in this. The initial platform gives you some basic summary statistics about how many single word terms have been identified, the number of rows, a list of the single word terms listed by frequency (for example, ‘pilot’ is very common, as is ‘during’, ‘maintain’ and ‘factors’). Each of these documents consists of words which are sequences of characters that don’t have white space between them. We take the words, and multiple sets of words form phrases. For example, ‘maintain directional control’, ‘failure to maintain’ and so on. What we’re trying to do is take this and create an indicator matrix – basically a design matrix for the text – that is going to have a column for ‘pilot’. This column will be marked ‘0’ for every document in which it is not present, or ‘1’ for every document in which it is. We’ll do this for all the terms we think are worth considering, and then, based on this big matrix, we’ll apply multivariate methodology.
After the initial process of feature creation is the process of identifying the terms and phrases that we want to use. Some of the original words we’re going to throw overboard because we don’t want to pay attention to them because they’re not useful to us. And some phrases we’ll want to treat as ‘terms’ – a ‘term’ is really a column in this design matrix – for that phrase. ‘Maintain directional control’ sounds pretty important, so we probably want to keep track of that as a unique identity independent of the separate words ‘maintain’, ‘directional’ and ‘control’. What we’ve provided in JMP 13 is a lot of tools for organising this. We might look at the word ‘failure’ – it’s pretty important! – and select ‘Containing Phrases’ and it will tell us which phrases contain the word ‘failure’ in them. We might decide that these are significant enough that we want to consider them within our document term matrix, so I’d click ‘Add Phrase’, and they will be added to our ‘terms list’. At the bottom of this list, we have a bunch of phrases that only occur once – they’re probably not worth paying much attention to; it’ll just muck up our analysis by keeping track of all of these. So you can select all the relatively low incidence terms and throw them overboard. Being arbitrary, I’d say anything that’s 20 incidences or below can be thrown out. You remove that term from consideration by calling it a ‘stop word’. Now all the terms that are in this list are the ones that will be added to the matrix.
Another thing that’s good to do is called stemming. Stemming is the process of treating like words; ‘pilot’, ‘pilots’, ‘piloting’, ‘piloted’, all as the same concept. Those will all get mapped into the same design matrix row. If any of those terms are present, the indicator for that stemmed term will be equal to ‘1’. We’ve collapsed all those words into one concept. This can be done really easily within JMP 13.
There’s also the ability to do some recoding. We can recode misspellings, for example. If you see something that is misspelt, often you can replace it. For example, if it’s the word ‘cat’ and you see a lot of ‘ct’ in your document, you can replace ‘ct’ with ‘cat’, thus changing that word in the representation of the document that is local to the platform, while leaving it unchanged in the original data table.
Chris Gotwalt on... stemming and re-coding if you’re creating your own custom sets and where they're held:
We have management resources; there’s the built-in set of ‘stop words’. You have a base list of words which are automatically treated as a ‘stop word’, like ‘a’, ‘an’, ‘the’ – those aren’t really helpful. This list will also include all the words you’ve thrown overboard, as I’ve previously mentioned. I could export this list into a user column, or I could use it to create a table or export it as a file. You can build it, use it, and re-use it later on.
Chris Gotwalt on... Text Explorer handling Regular Expressions:
We have a great Regular Expressions utility. We have a bunch of tools built in, so – for example – what it will do is identify the terms that are ‘money’ and have it replace that with whatever you like. You could have it be ‘£’ money, so that whenever you have a match for this regular expression in the description of money, it’ll map it to the word ‘£’, so that it stems ‘money’ into one concept. It’s a fully supported regular expression process and text replacement tool. Just go to the Regular-Expressions website, figure out how to get a regular expression that does what you need added, and then create it… then I normally forget all about it. That’s something you’d definitely want to save to your custom set of regular expressions… because once you’ve done it once, you won’t want to do it again!
If you're interested in receiving details of future events, please contact us. Be the first to know about new blogs, upcoming courses, events, news and offers by joining our mailing list here.